MIEP Applies Supervised Machine Learning Methods to Accelerate Immunology Research
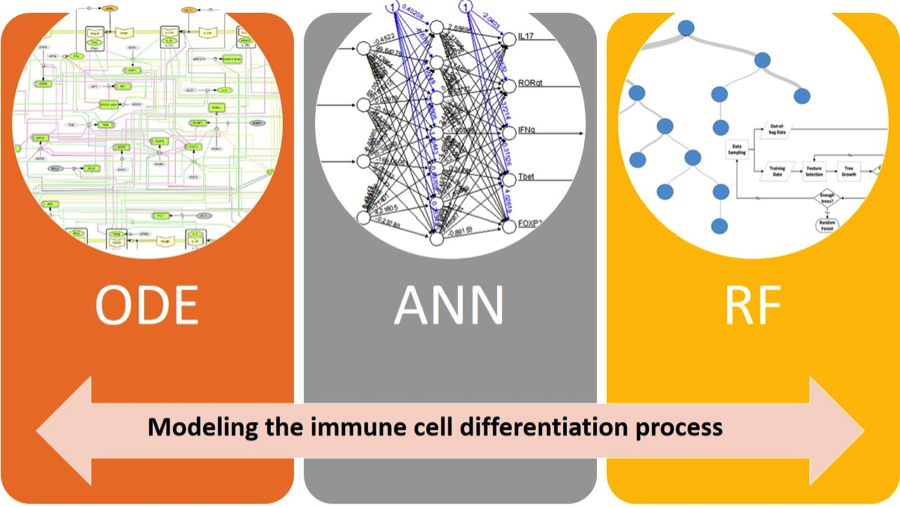
Novel data analytic approaches are required for modeling immune non-linear and complex immune responses. Each cell type of immune system is highly diverse and can be further differentiated into subsets with unique and overlapping functions. To study cellular and molecular mechanisms of cell differentiation, computational systems biology approaches can provide information-processing representations of these immunological processes. Even though equation-based and agent-based modeling (ABM) approaches and have been successful in simulating complex biological behaviors, building such complex models requires substantial effort, resources, and significant support from domain experts in the field.
The MIEP team successfully performed the first study deploying supervised learning methods to model immune cell differentiation. This study compares four supervised learning methods for modeling CD4+ T cell differentiation: Artificial Neural Networks (ANN), Random Forest (RF), Support Vector Machines (SVM), and Linear Regression (LR). Artificial Neural Networks and Random Forest models showed comparable results and outperform the two other widely used methods (LR and SVM). This work has been published in Biodata Mining journal.
The ANN and RF models were also robust to noise and showed comparable results to the detailed equation-based model; these models were also able to reproduce the results from three published studies. Furthermore, ANN and RF models represent ideal candidates for integration into the agent-based models that we have developed using ENISI MSM to study the immunological processes comprehensively and systematically. Using machine learning algorithms in combination with ODE- and ABM-based methods will reduce the computational complexity of the system and allow us to uncover layers of hidden knowledge in immune responses that can lead to a deeper systems-wide understanding of the complex interplay between molecules, cells and tissues of the immune system. Altogether these methods will ultimately advance the development of safer and more efficacious therapeutics for infectious and immune-mediated diseases.
About NIMML
The NIMML Institute is a 501 (c) (3) non-profit public charity foundation focused on a transdisciplinary, team-science approach to precision medicine at the interface of immunology, inflammation, and metabolism. The NIMML Institute team has led numerous large-scale transdisciplinary projects and is dedicated to solving important societal problems by combining the expertise of immunologists, computational biologists, toxicologists, modelers, translational researchers, and molecular biologists. The Institute is headquartered in Blacksburg, VA. For more information, please visit www.nimml.org or contact pio@nimml.org.